By Shrikant Dash and Richard Pieters The authors each have about three decades of experience in industrial and service businesses like GE, DSM, Zymergen, Discover, and Citi, both in the deployment of six sigma as well as advanced analytics to improve operations, and scale and grow businesses.
Introduction
The last few years have seen a proliferation in data infrastructure and flexible computing environments, as well advances in multiple machine learning algorithms that provide robust outcomes, particularly with high-frequency, unstructured, and non-numerical data (images, language, social media perceptions). This presents an opportunity for firms to invest in AI enabled processes that drive a disproportionately high level of automation efficiency and control in manufacturing processes aligned with customer preferences and experience. However, since the data fabric/AI tools landscape is rapidly evolving and is fragmented, the investment decisions are fraught with risks.
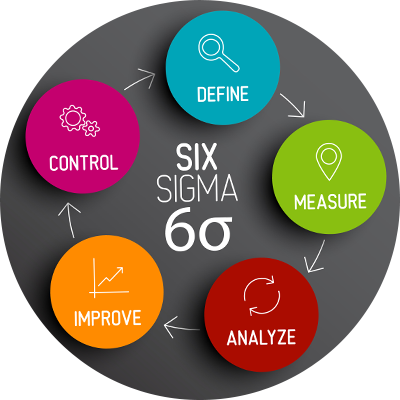
We highlight six sigma/lean manufacturing techniques, based on our experience with change management in industrial and services businesses in the last 30 years, as a valuable lens for the planning and execution of AI adoption and investment roadmaps, by seeking to reduce financial as well as operational risks.
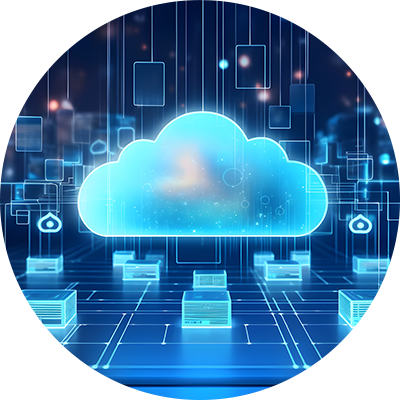
The AI-enabled Enterprise
Manufacturing, as well as service companies, always seek to allocate capital efficiently and deploy it to maximize customer and shareholder value, and, with the advent of richer data environments, AI and machine learning tools, and deeper, more predictive customer insights, there is an opportunity for early adapters to build agility and efficiency and reduce control failures while creating focused business value. As we focus on the leadership perspective here, we need to consider business and product strategy as key target areas for improved decision making. Information (which is data plus meaning) can make leaders understand better the competitive landscape, enhanced customer insights that are not expressed clearly, the impact of megatrends, and the wellbeing of their current business. Also, inefficiencies and operational improvements can be analyzed with the right kinds of information.
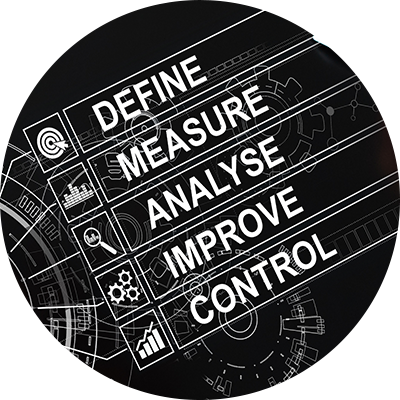
The Role of Six Sigma/Lean Manufacturing Principles
Six Sigma is a way of thinking about business processes from the standpoint of continuous improvement. It uses techniques to measure and reduce “variance” in relevant KPIs (key performance indicators) so that these processes are not wasteful, and continually enhance customer value, and therefore promote profitable growth. Lean manufacturing principles extend that way of thinking by focusing on agility (speed-to-market) and process connectivity. Looking at the business process as an inverted supply chain from the standpoint of customers, this outside-in approach seeks to Define/Measure/Analyze/Improve/Control in the now well understood DMAIC framework used by Motorola, GE, and a host of other industrial and service firms.
It is obvious that the six sigma/lean manufacturing approach is data-driven and in a value-focused way. In the following, we would recommend this process-oriented approach to the strategic adoption of, and investments in, data infrastructure, AI tools, and human capital as well as change management processes. Foundational to all this is an appropriate investment in data infrastructure, particularly modified to incorporate newer, higher frequency digital and qualitative data, as well as data visualization and BI tools, and more elastic computational environments and algorithm sets that can work in areas such as deep learning, generative AI, and non-linear prediction.
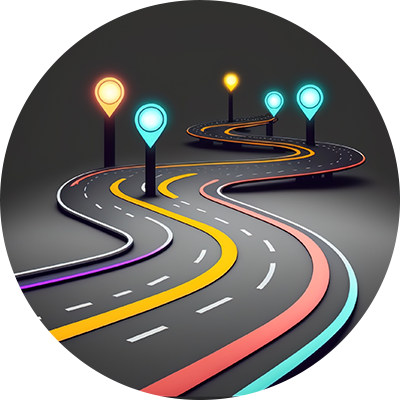
A Six Sigma Roadmap for AI Adoption
In building a rapid adoption roadmap for AI enable enterprises, the following are a set of key factors that should be considered:
- What industry domain are you in? Is the business data rich or data poor? Think of brick-and-mortar vs. digital enterprises/B2B vs. B2C businesses [Define]
- What is the frequency quality and type of data that is being generated while conducting business? Are we collecting all the relevant data that is critical to quality? Is it stored and used to improve business processes efficiently and effectively? [Define/Measure]
- What are the key sources of competitive market value from the point of view of our customers? Are we considering all customer insights in seeking operational improvements? What are the critical business drivers of profit, growth, and customer value? Do we have accurate measurements for those critical KPIs? Are those measurements visible to all key stakeholders on an ongoing basis? [Define/Measure/Analyze]
- Is our data/information tool stack optimized around key drivers of business value? Do we have the right investments in insight generation and business intelligence? Are measurements and data elements standardized, with good metadata? Is access to stakeholders easy, and are reporting/insight lags minimized? [Measure/Improve]
- Do we have the right human capital to use the information stack? Are they knowledgeable about evolving AI and machine learning approaches? Are they properly organized at the right level of organizational seniority/visibility? Are the key business leaders and stakeholders properly incentivized to deliver business value based on AI adoption, or is this the “flavor of the month”? [Improve/Control]
- Have we defined key success factors/do we know what WIN looks like? Do we have the right tracking metrics for investments, adoption, and value delivery related to AI? What processes do we have to course correct/what are the fast failure modes? How do we document/memorialize AI adoption tollgates via reporting and reviews? How do we achieve scalable and sustainable value from AI? [Improve/Control]
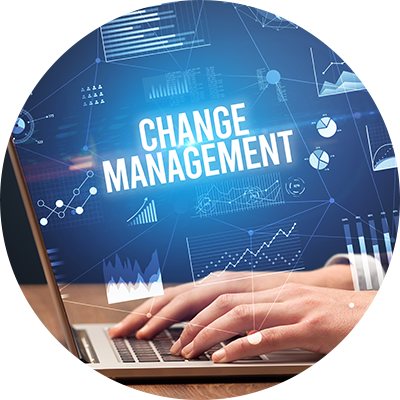
AI Capability Building & Change Management
In the six-sigma approach, a key success factor is the emphasis on change management. Educating people across the enterprise on the role of data and analytics, empowering them through ongoing training, celebrating adoption, are all critical:
- Relate AI adoption/investments clearly to business and individual incentives
- Organization Education, language, and cultural acceptance (Transformation)
- Address business maturity in using data-driven insights (cultural issues)
- Examine and shut down legacy processes that are manual/judgmental
- Invest in a senior business minded analytics leader
- Identify and celebrate quick wins through AI adoption
- Quantify and publicize business results that are due to AI
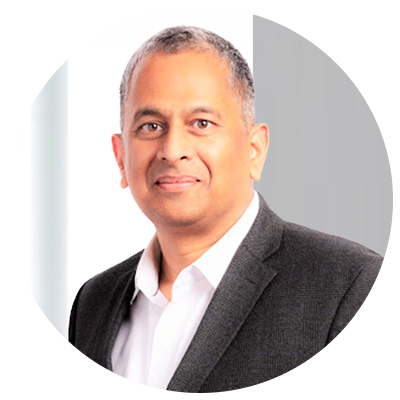
Shrikant Dash
Managing Partner

These market insights from Carpe Diem Global Partners are gathered from the firm’s extensive client work leading Board, CEO, CXO, and CHRO executive search engagements for public and private multinational companies. For deeper, custom insights, contact Shrikant Dash at sdash@carpediempartners.com or Richard Pieters at richard.pieters@live.nl.
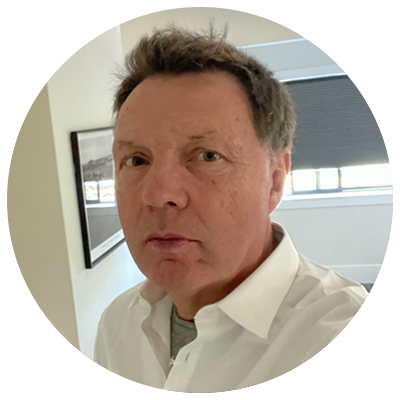
Richard Pieters
CEO / Advisor