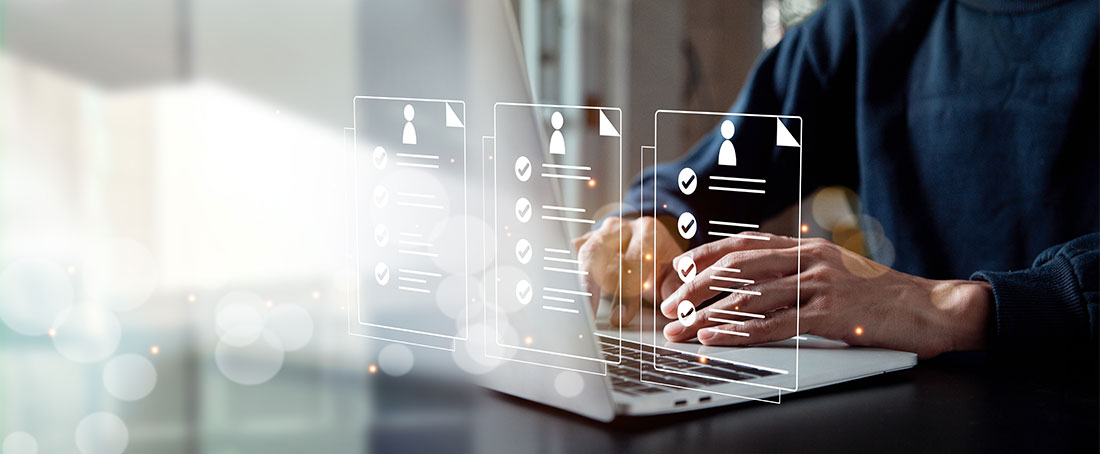
II. Employee Leadership Assessment
IDC’s Future of Work 2022 research predicted that this year, 60 percent of global 2000 businesses will deploy AI and machine learning (ML) tools to support the entire employee life cycle experience. By 2024, the authors predict, 80 percent of the global 2000 organizations will use AI/ML-enabled “managers” to hire, fire and train employees. In publicly traded companies, evaluating leadership performance has become an increasingly complex, data-driven endeavor. AI/ML systems offer comprehensive analyses of leadership effectiveness, surpassing traditional methods. They can scrutinize large datasets, including financial performance metrics and market trends, to evaluate the impact of executive behaviors and decisions impact on company performance. By integrating data from diverse sources, AI presents a holistic view of a leader’s influence on the company’s market reputation and brand value.
Just as in the acquisition of talent, it is important to treat employee assessment and development as a continuous process rather than as a series of disconnected events. The mapping of employee journeys over their entire organizational tenure, from onboarding and role changes as well as promotional roles, ending in exit interviews, from the point of view of information management, is an invaluable dataset for predictive and prescriptive analytics of human capital. When an organization systematically invests in a comprehensive and structured store of information about its human capital, it can better understand motivations for performance, rationale for executive decisions, as well as group dynamics within the organization.
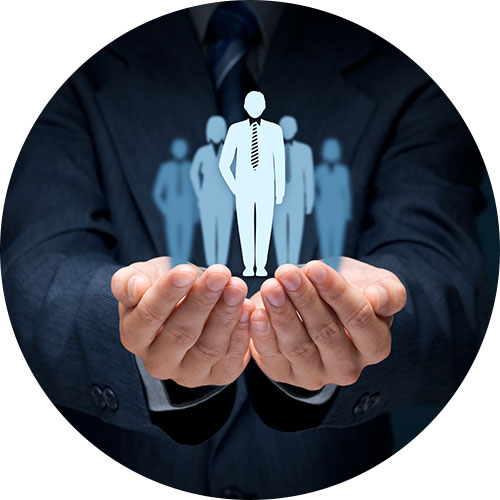
There is a slight nuance in the types of data that are more predictive of leadership success in group decision-making when one is evaluating internal talent versus talent acquisition. The fit with role requirements, prior experience, skills, and educational qualifications are likely to be stronger predictors in AI based algorithms of external talent assessment. These can be enhanced by references but may be subject to various kinds of bias. On the other hand, for internal leadership talent, the wealth of internal behavior assessments and prior evaluations of collaboration, influence, thought leadership, followership, and culture fit, encapsulated in 360-degree feedback, can be powerful predictors of employee success in future leadership roles.
A key strength of AI/ML in leadership assessment is its ability to process unstructured data, such as employee feedback and leadership communication styles, enabling the evaluation of qualitative aspects of leadership. AI algorithms also benchmark leadership performance against industry standards, providing insights into competitive positioning and strategic effectiveness. Predictive analytics can forecast future trends in leadership, aiding in succession planning.
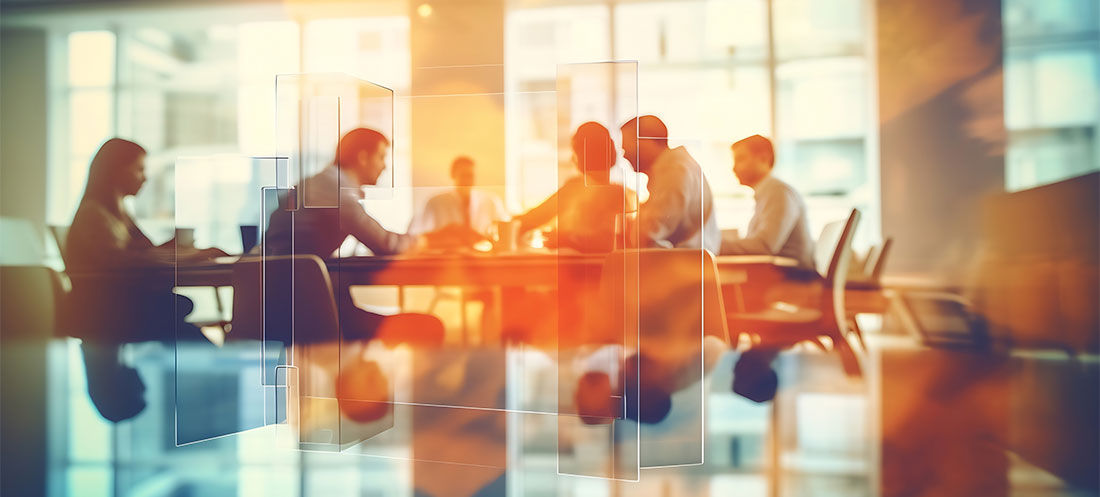
Once an organization has invested in creating human capital data and information management platforms, as many are beginning to do, then AI and ML techniques can be deployed both at an individual and functional level, as well at the level of the enterprise. For example, 360-degree feedback can be summarized efficiently as well as effectively using generative AI both to reduce cycle time in the evaluation process and to reduce evaluation bias and present the information more effectively by standardizing input paraments across evaluators. Similarly, AI and ML techniques are used in leadership interactions in virtual gamified settings and in training modules that iteratively tease out relative strengths and weaknesses.
At an enterprise level, business intelligence and data visualization techniques have become increasingly common in the creation of digital human capital dashboards in areas such as training, succession planning, diversity and inclusion, (high potential) employee attrition, and time-in-role/promotions/cross-functional movement metrics. These dashboards often highlight gaps in organizational achievement in these areas and areas where industry-leading success has been achieved. This data can also be used to signal the quality of human capital management as “employer of choice” to compete in the external war for talent.
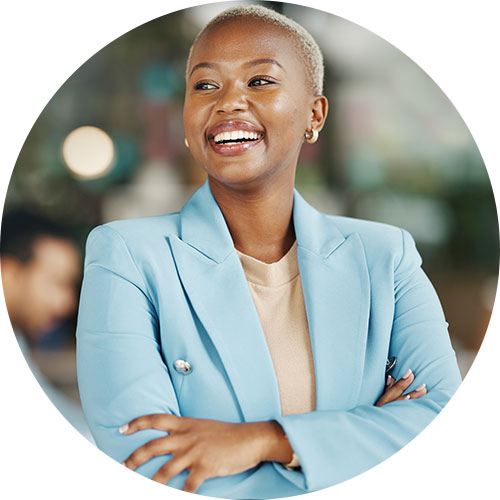
In summary, the ability to collect and use human capital data has increasingly created the possibility to effectively manage leadership talent evaluation and assessment as a process and journey, rather than as a series of disconnected events. In the future, the proliferation of predictive AI tools and evaluation instruments will take the guesswork out of human capital management.
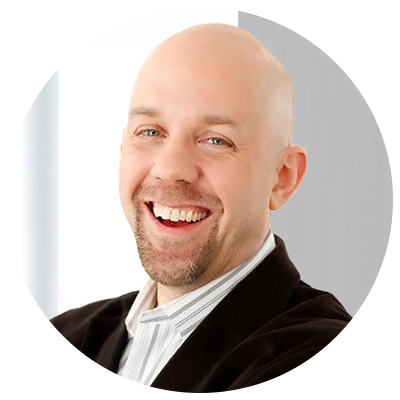
Matthew Roberts Managing Partner

These market insights from Carpe Diem Global Partners are gathered from the firm’s extensive client work leading Board, CEO, CXO, and CHRO executive search engagements for public and private multinational companies. For deeper, custom insights, contact Matthew Roberts at mroberts@carpediempartners.com or Shrikant Dash at sdash@carpediempartners.com .
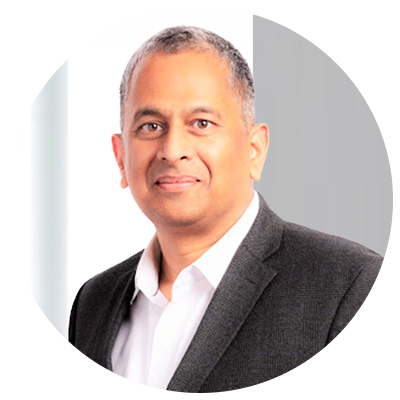
Shrikant Dash
Managing Partner